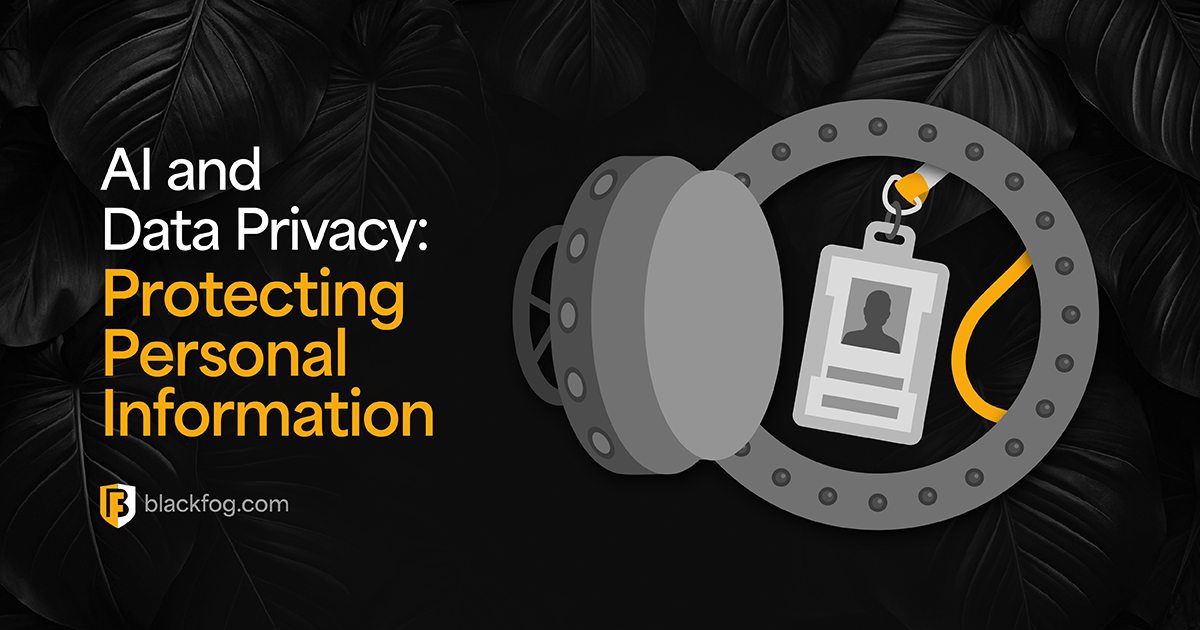
AI and Data Privacy: Protecting Personal Information
Artificial intelligence is revolutionizing the way businesses collect, process and analyze data. But as AI systems become more sophisticated, they are handling ever-growing volumes of personal and sensitive information. This raises serious concerns about data privacy, security, and compliance.
While AI offers huge potential for automation, threat detection and business intelligence, it also presents new risks. So what can businesses do to balance the power of AI against the need for data privacy?
AI’s Growing Role in Data Privacy
AI is playing an increasing role in how businesses manage their data. This presents both opportunities and threats. Firms that are able to take full advantage of these capabilities will find they have access to much greater insight into their operations and their customers. However, at the same time, the increasing volumes of highly sensitive data processed using these tools will present tempting targets for hackers.
There will also be a growing number of compliance regulations that must be adhered to when deploying AI. These will cover what data is collected, how this is used and who will be authorized to access it.
All new technologies that handle sensitive data will present cybersecurity and privacy challenges. However, AI is different because it has the potential to analyze vast quantities of data, learn and adapt to its inputs, and then make potentially business critical decisions without clear, transparent processes or oversight.
The Intersection of AI and Data Privacy
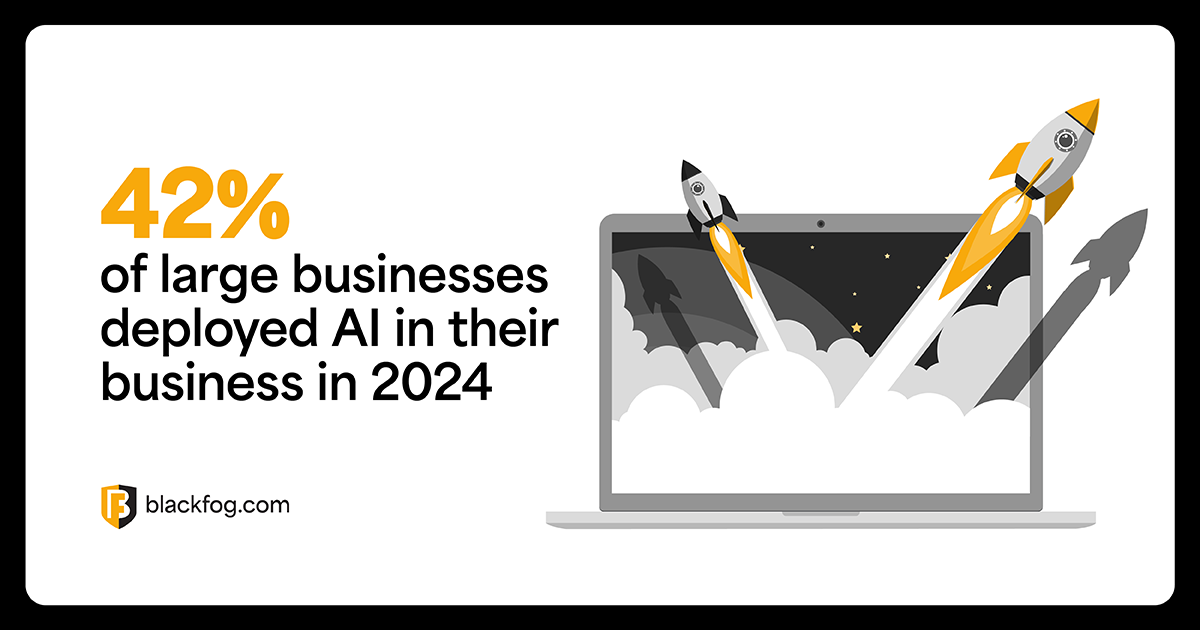
AI-driven tools already interact with large amounts of personal and business data. In the coming years, the volume of information used by these systems is only set to increase even further.Â
For instance, one study by IBM in 2024 found 42 percent of large businesses (those with over 1,000 employees) had already deployed AI in their business, while a further 40 percent were exploring the technology. The most common applications were:
- Automation of IT processes (33 percent)
- Security and threat detection (26 percent)
- AI monitoring or governance (25 percent)Â
- Business analytics or intelligence (24 percent)Â
- Automating processing, understanding and flow of documents (24 percent)
Some examples of how AI-driven systems collect and use data include:
- Web activity monitoring
- Location tracking
- Facial recognition
- Social media monitoring
All of these involve highly personal data that offers the potential for abuse by unethical users or external hackers.
There have already been several real-world cases where the use of AI was involved in data privacy breaches, whether intentionally by hackers or as the result of mistakes in the business.
For example, in 2023, Samsung accidentally leaked sensitive information including trade secrets after employees turned to ChatGPT for help with writing code, uploading private documentation to the third party in the process. Amazon has also warned employees not to share confidential data with the platform after similar incidents.
Hackers have been able to use techniques such as prompt injection to trick AI platforms into handing over sensitive information. This makes AI another front in the fight against data exfiltration.Â
Key Privacy Challenges in AI
IBM’s 2024 study found that challenges of privacy and transparency are particularly important when businesses are deploying generative AI tools. Nearly six out of ten IT pros (57 percent) named privacy concerns as a barrier to entry, while 43 percent expressed worries about transparency.
However, these are just some of the key challenges facing businesses when it comes to letting AI loose on their most sensitive data. Some of the more specific threats that will need to be addressed include:
- Unauthorized collection of data: It can be easy for indiscriminate AI to collect data without the explicit consent of users, which will be a breach of privacy regulations. Many AI applications gather data through tracking cookies, voice assistants, and social media interactions, with little user awareness or oversight.
- Managing highly sensitive information: Highly sensitive information such as biometrics needs particularly careful attention when being used in AI. As well as issues of consent, misuse of this data to create deepfakes poses new privacy and security challenges.
- AI-driven surveillance: The ability to track users through tools like facial recognition or monitoring browsing activity can give huge insight into customer behavior and habits, but can also easily intrude into users’ expectations of privacy.
- Lack of transparency in decision-making: Many AI systems use reasoning and decision-making processes that even their creators do not fully understand. This can lead to distrust among users and make it hard to explain why certain actions are being taken. From a privacy standpoint, it can be difficult to know if data is being used in accordance with regulatory and ethical considerations.
- Potential for data breaches: Cybersecurity must be a top priority for any business building AI systems. Due to the vast datasets these solutions require, they are a prime target for attacks. This is particularly true for those that use financial details, health records and biometric data. As such, it’s vital that AI solutions are closely integrated with key security solutions such as access controls, usage permissions and anti data exfiltration (ADX).
Regulations Governing AI and Data Privacy
Data privacy regulations such as the General Data Protection Regulation (GDPR) and the California Consumer Privacy Act (CCPA) set strict guidelines on how companies must handle personal data.
GDPR, enforced for any firms that process the data of EU citizens, mandates transparency, consent, and the right to be forgotten, requiring businesses to implement data protection by design. Similarly, the CCPA, applicable to companies handling California residents’ data, grants individuals rights to access, delete, and opt out of data sales. This applies as much to AI-driven processes as other forms of data usage.
Firms that fail to meet their obligations under these acts run the risks of large penalties. GDPR, for instance, allows regulators to fine companies up to €20 million or four percent of global turnover – whichever is larger. Some of the largest fines issued under GDPR so far, to the likes of Meta, relate to the mishandling of consumer data, so this is clearly a top priority for regulators.
To maintain compliance, firms must ensure algorithmic transparency, ethical data usage and cybersecurity safeguards. Businesses should adopt privacy-enhancing technologies (PETs), conduct regular data audits and implement AI ethics frameworks to assess potential biases and risks.Â
Encryption, data anonymization and robust access controls are essential measures to safeguard consumer data. By prioritizing compliance-first AI systems, organizations can mitigate legal risks while maintaining consumer trust in an era of heightened data security concerns.
Strategies for Protecting Data Privacy in the AI Era
With AI playing an increasing role in data processing, firms must adopt proactive strategies to comply with regulations and protect user privacy. Effective data privacy measures not only mitigate risks but also build trust with customers and stakeholders. The following strategies are essential for ensuring AI-driven systems handle personal data responsibly.
Implementing Privacy-by-Design Principles
Privacy should be integrated into AI systems from the outset, not as an afterthought. Firms must design AI processes that limit data exposure by using techniques such as data anonymization and access controls. Regular risk assessments and automated privacy checks should be embedded into development cycles to proactively identify and mitigate vulnerabilities.
Enhancing AI Transparency and Explainability
Some AI models can obscure how data is used, raising compliance and ethical concerns. Businesses should adopt interpretable models and provide clear documentation on data processing to avoid this. There are tools available that can assist with this, such as SHAP (Shapley Additive Explanations) and LIME (Local Interpretable Model-Agnostic Explanations). These both aim to offer clearer explanations of how decisions have been made, which helps ensure AI decisions are auditable. Firms should also maintain public AI usage policies to demonstrate accountability and build user confidence.
Using Encryption and Secure AI Models
Data security is paramount when handling sensitive information. This is particularly true when utilizing cloud-based third-party AI models that require firms to send sensitive information outside their network perimeter. Companies must implement end-to-end encryption for data in transit and at rest, ensuring AI models do not inadvertently expose personal data. Adopting techniques that allow AI to process data without decryption, such as homomorphic encryption, also reduces the risk of data being intercepted by hackers or inadvertently published.
Minimizing Data Collection and Retention
It can be very easy for large-scale generative AI tools to ingest huge amounts of data, of which only small portions may actually be relevant. Companies may also unintentionally collect sensitive data they do not have consent to use. To avoid this, firms should establish exactly what type of data their models will need and then enact data minimization policies. Automated data retention and deletion processes should also be enforced, identifying what data needs to be kept and removing all other information after its intended use.
AI’s Role in Strengthening Data Security
While AI does present a range of data privacy challenges for businesses, it can also greatly enhance security and provide better data protection for firms handling sensitive information. If cybercriminals are able to gain access to sensitive information and exfiltrate data for ransomware extortion, the damage done can be considerable.Â
As well as the direct financial costs associated with recovering from a data breach, there are severe reputational and regulatory issues to contend with. Consumers are more aware than ever of the value of their data and will be unwilling to do business with companies that mishandle information or expose it to criminals. Regulators, meanwhile, can also levy large fines for poor data protection practices that allow a breach.
AI can assist with this in several ways. Among the most common applications for the technology in data security are:
- Network monitoring: AI can learn what normal activity looks like and then watch for subtle changes in user behavior, which may indicate a hack in progress.
- Threat detection: Smart threat detection can also look beyond traditional signatures for patterns of activity such as copying or editing files. This can be used to track down adaptive malware that actively changes its code in order to evade detection.
- Automated incident response: These tools can conduct risk assessments on any anomalies they find and then determine the best course of action to shut down any attacks without needing to ask a human operator for instructions.
The Future of AI and Data Privacy: Balancing AI Innovation and Protection
AI and privacy issues will continue to be closely linked in the coming years. With these technologies set to become commonplace in many aspects of business, consumers and regulators will place increasing demands on firms to make privacy a top priority.
Firms will be expected to have clear ethics in place for their use of AI, with frameworks that emphasize the importance of transparency and accountability. Such programs will be essential against a backdrop of more stringent regulations for the use of the technology, such as the EU’s AI Act, which is being gradually rolled out from 2025 onwards.
AI data privacy solutions will need to strike a delicate balance between the powerful capabilities of AI to analyze data and make decisions, and the need for user privacy to be respected. Businesses that prioritize transparency, security, and compliance will not only meet regulatory demands, but also build a competitive edge.
Related Posts
Black Basta Ransomware: Protection, Prevention, and Recovery Guide
Learn how to stay safe from Black Basta ransomware group with advice on how to spot, prevent, and recover from attacks. Understand how these attacks work, explore real-life examples, and discover strategies to protect against ransomware.
Ascension Ransomware Attack: Impact and Prevention Tips
Learn how the Ascension ransomware attack disrupted healthcare services, the financial consequences, and the cybersecurity lessons it taught. Also receive advice on protecting patient data and preventing similar attacks in the future.
Essential Data Loss Prevention Best Practices Every Firm Should Know
Following these seven data loss prevention best practices can help any firm reduce the risk of falling victim to threats like ransomware.
BlackFog Report Reveals Record Number of Ransomware Attacks from January to March
BlackFog reports a record-breaking surge in ransomware attacks Q1 2025, with 278 disclosed cases and a 113% rise in undisclosed incidents.
AI for Network Security and Monitoring: Enhancing Cyber Defense
What opportunities do AI ransomware protection tools offer to cybersecurity pros?
Ghost Ransomware: The New Cyber Menace Targeting 70+ Countries
Ghost ransomware is targeting 70+ countries. Learn how it works, who’s behind it, and how to stay protected in today’s cyber threat landscape.